Table of Contents
Predictive maintenance is revolutionizing the way industries manage and maintain their equipment. By leveraging artificial intelligence (AI), businesses can anticipate equipment failures before they occur, thereby reducing downtime, extending the lifespan of machinery, and improving operational efficiency. AI-driven predictive maintenance represents a significant advancement from traditional maintenance strategies, offering a data-driven approach to keeping operations running smoothly.
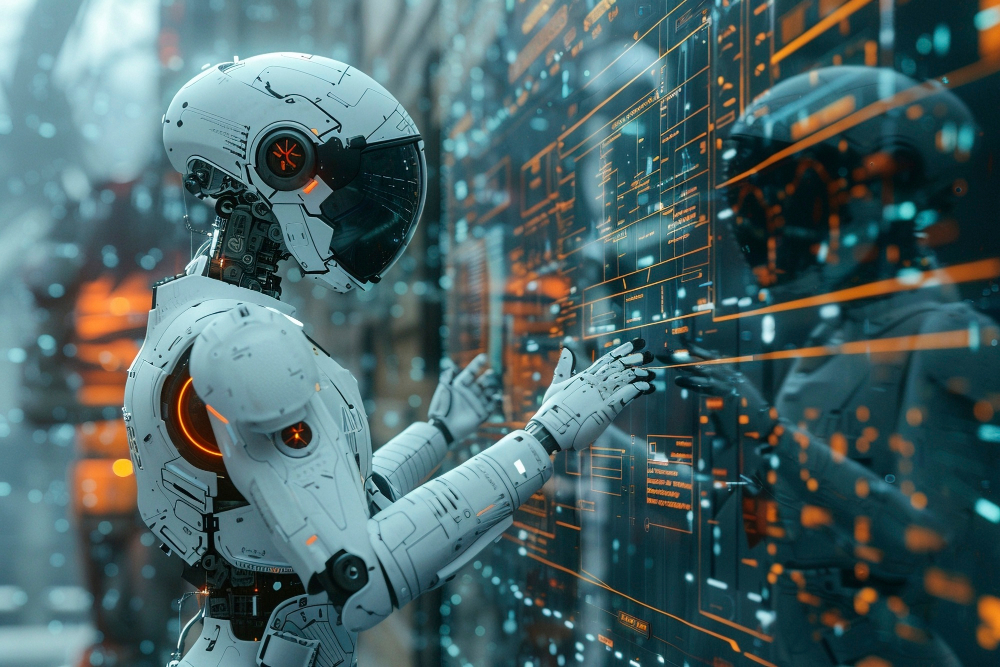
Understanding Predictive Maintenance
Predictive maintenance involves using data and analytics to predict when equipment is likely to fail so that maintenance can be performed just in time to prevent unexpected breakdowns. Unlike reactive maintenance, which addresses issues after they arise, or preventive maintenance, which schedules regular maintenance based on time intervals, predictive maintenance relies on real-time data and sophisticated algorithms to make informed predictions.
Traditional predictive maintenance methods often rely on historical data and statistical models. However, with the advent of AI, the approach has become more advanced, utilizing machine learning, data mining, and other AI techniques to analyze vast amounts of data and provide more accurate forecasts.
How AI Enhances Predictive Maintenance
- Real-Time Data Analysis
AI excels in processing and analyzing large volumes of data in real-time. In the context of predictive maintenance, AI algorithms can continuously monitor equipment performance through sensors and IoT devices. This real-time data is fed into machine learning models that identify patterns and anomalies indicative of potential failures.
For example, AI can analyze vibrations, temperature, pressure, and other operational parameters to detect deviations from normal operating conditions. By recognizing these anomalies early, AI systems can predict equipment issues before they escalate, allowing for timely maintenance and preventing costly breakdowns.
- Machine Learning and Anomaly Detection
Machine learning algorithms are at the heart of AI-driven predictive maintenance. These algorithms are trained on historical data to recognize patterns and predict future behavior. As new data is collected, the algorithms continuously learn and adapt, improving their accuracy over time.
Anomaly detection is a critical component of predictive maintenance. AI systems use machine learning models to identify unusual patterns or outliers in equipment data. For instance, if a motor’s vibration levels suddenly increase beyond established norms, the AI system can flag this as a potential issue, prompting further investigation and maintenance.
- Predictive Analytics and Forecasting
AI-powered predictive analytics provide valuable insights into equipment health and performance. By analyzing historical data and current conditions, AI models can forecast when maintenance will be required. These forecasts are based on a combination of factors, including wear and tear, usage patterns, and environmental conditions.
Predictive analytics enable businesses to optimize maintenance schedules, balancing the need for preventive maintenance with the goal of minimizing operational disruptions. By forecasting potential failures, organizations can plan maintenance activities during scheduled downtime, reducing the impact on production and ensuring that equipment remains in peak condition.
- Optimization of Maintenance Resources
AI-driven predictive maintenance helps optimize the allocation of maintenance resources. By accurately predicting when and where maintenance will be needed, organizations can allocate personnel, tools, and spare parts more efficiently. This targeted approach reduces the risk of over-maintenance or under-maintenance, leading to cost savings and improved resource utilization.
For example, if AI predicts that a specific piece of equipment will require maintenance in the near future, the organization can schedule the necessary technicians and parts in advance. This proactive approach minimizes disruptions and ensures that maintenance activities are carried out efficiently.
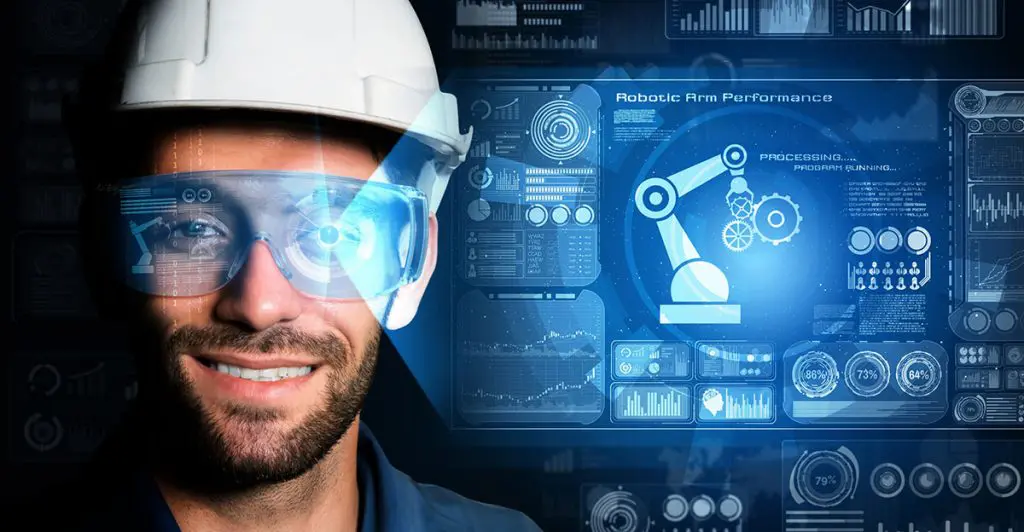
- Enhanced Decision-Making
AI enhances decision-making by providing actionable insights and recommendations based on data analysis. Predictive maintenance systems can generate reports and visualizations that help maintenance teams understand equipment health, performance trends, and potential issues.
These insights empower decision-makers to prioritize maintenance activities, allocate resources effectively, and make informed choices about equipment replacement or upgrades. By leveraging AI-generated recommendations, organizations can improve their overall maintenance strategy and achieve better operational outcomes.
- Integration with IoT and Digital Twins
AI’s role in predictive maintenance is closely linked with the Internet of Things (IoT) and digital twins. IoT devices collect real-time data from equipment, which is then analyzed by AI algorithms. Digital twins, which are virtual replicas of physical assets, provide a dynamic model of equipment performance and behavior.
By integrating AI with IoT and digital twins, organizations can create a comprehensive view of equipment health and performance. This integration allows for more accurate predictions and simulations, enabling maintenance teams to test different scenarios and assess the impact of various maintenance strategies.
- Continuous Improvement and Learning
One of the key benefits of AI in predictive maintenance is its ability to continuously improve and learn. As more data is collected and analyzed, AI models become increasingly accurate and effective. This continuous learning process allows predictive maintenance systems to adapt to changing conditions and refine their predictions over time.
Organizations can leverage this continuous improvement to enhance their maintenance practices, identify emerging trends, and address new challenges. By staying ahead of potential issues and incorporating feedback from maintenance activities, businesses can further optimize their predictive maintenance strategies.
Challenges and Considerations
While AI offers significant advantages for predictive maintenance, there are challenges to consider. Implementing AI-driven systems requires substantial investments in technology, data infrastructure, and expertise. Additionally, the quality and accuracy of predictions depend on the availability and reliability of data.
Data privacy and security are also important considerations, as the collection and analysis of equipment data involve sensitive information. Organizations must ensure that their AI systems comply with relevant regulations and protect data from unauthorized access.
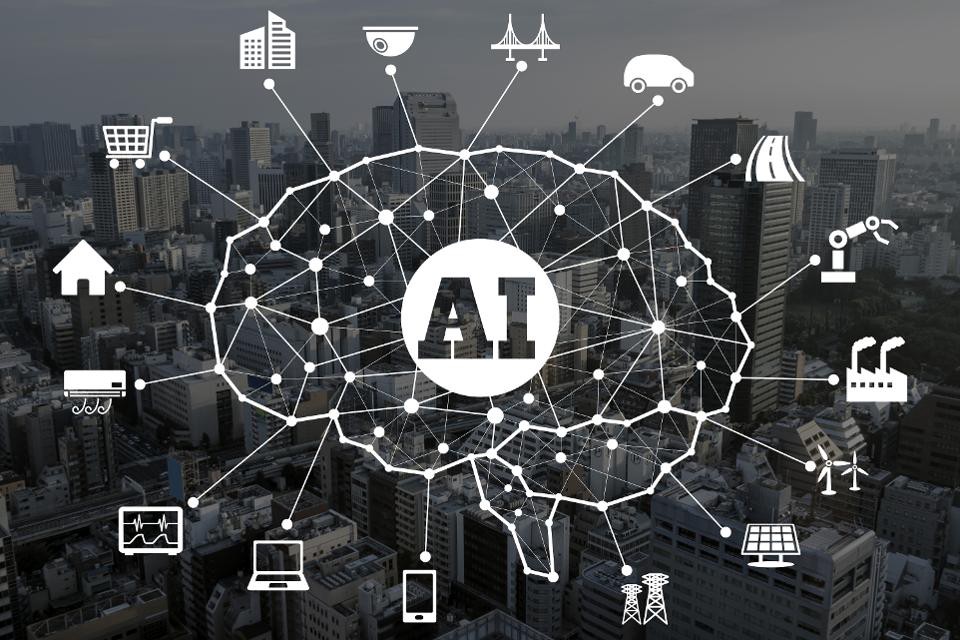
Conclusion
AI is transforming predictive maintenance by providing advanced tools and techniques for anticipating equipment failures and optimizing maintenance activities. Through real-time data analysis, machine learning, predictive analytics, and integration with IoT and digital twins, AI enhances the accuracy and efficiency of maintenance practices. While there are challenges to address, the benefits of AI-driven predictive maintenance—such as reduced downtime, cost savings, and improved operational efficiency—make it a valuable asset for modern industries. Embracing AI technology in predictive maintenance can lead to more reliable operations and a competitive edge in an increasingly complex and data-driven world.